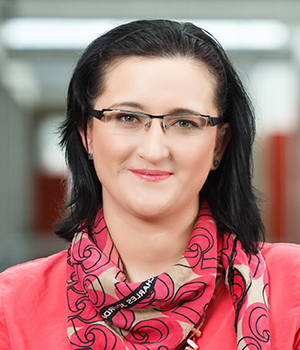
Marinka Zitnik, PhD
Marinka Zitnik is an Associate Professor in the Department of Biomedical Informatics at Harvard Medical School, Associate Faculty at the Kempner Institute for the Study of Natural and Artificial Intelligence at Harvard University, Associate Member at the Broad Institute of MIT and Harvard, and Affiliated Faculty at the Harvard Data Science Initiative.
Artificial intelligence is poised to enable breakthroughs in science and reshape medicine. Dr. Zitnik investigates foundations of AI to enhance scientific discovery and to realize individualized diagnosis and treatment. The overarching goal of her lab is to lay the foundations for AI that contribute to the scientific understanding of therapeutic design and genomic medicine or acquire such understanding autonomously.
- AI for Medicine: The state of a person is described with increasing precision incorporating modalities like genetic code, cellular atlases, molecular datasets, and therapeutics—the challenge is how to reason over these data to develop powerful disease diagnostics and empower new kinds of therapies. Our research creates new avenues for fusing knowledge and patient data to give the right patient the right treatment at the right time and have medicinal effects that are consistent from person to person and with results in the laboratory.
- AI for Science: For centuries, the method of discovery—the fundamental practice of science that scientists use to explain the natural world systematically and logically—has remained largely the same. We are using AI to change that. The natural world is interconnected, from the various facets of genome regulation to the molecular and organismal levels. These interactions across different levels yield a bewildering degree of complexity. Our research seeks to disentangle this complexity, developing AI models that advance drug design and help develop new kinds of therapies.
Her lab is pioneering AI systems informed by geometry, structure, and symmetry, grounded in scientific knowledge. Current research directions in the lab include:
- Large pre-trained AI to fuse data modalities like genetic code, single-cell atlases, molecular datasets, and therapeutics via multimodal knowledge graph networks and large pretrained language models
- Geometric deep learning and graph neural networks to reason about network biology and medicine
- Multi-scale, individualized, and contextualized AI to transfer prediction prowess acquired from one data type to another and to design contextually adaptive AI that can dynamically adjust outputs to biological contexts, such as patients, diseases, and cell types, in which they operate
- Better methods for drug design to enhance drug development across therapeutic modalities and stages of development
- Foundations for scientific discovery in the age of AI.
Dr. Zitnik has founded Therapeutics Data Commons and is the faculty lead of the International AI4Science initiative.
In 2020 she organized the National Symposium on Drug Repurposing for Future Pandemics on behalf of the National Science Foundation (NSF)
Current Postdoctoral Fellowship Opportunities
- Artificial Intelligence for Cancer Drug Discovery
- Representation learning and embeddings for biomedical networks and knowledge graphs
DBMI Research Areas
DBMI Courses
Cell Syst
View full abstract on Pubmed
Cell
View full abstract on Pubmed
ArXiv
View full abstract on Pubmed
Nat Med
View full abstract on Pubmed
Mol Pharm
View full abstract on Pubmed
medRxiv
View full abstract on Pubmed
Nat Methods
View full abstract on Pubmed
bioRxiv
View full abstract on Pubmed